GENIE: Intelligent Data Curation & Auto-Labeling for Efficient Data Labeling
Accelerating AI Training with Smart Data Labeling & Active Learning
Smart Labeling, 10X Faster
In the rapidly evolving landscape of machine learning (ML) and artificial intelligence (AI), GENIE stands as a transformative solution, designed by AvaWatz to streamline and optimize data preparation for AI training. By automating data labeling, active learning, and synthetic data generation, GENIE reduces manual effort, enhances dataset quality, and ensures AI models are trained on balanced, diverse, and high-fidelity data. This results in more accurate, reliable, and trustworthy ML models—significantly accelerating development cycles while improving performance across real-world applications.
Intelligent Data Processing Features
Active learning to prioritize critical data slices.
Automatic Labeling with Human Verification
Multi-Model Consensus & Weak Supervision
Synthetic Data Augmentation
Targeted Learning for Rare and Long-tail Classes
Data Filtering & Relevant Data Selection
CREATE HIGH CONFIDENCE AI MODELS
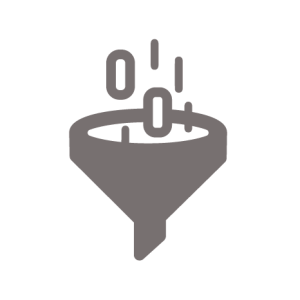
Relentless Refinement
Selects uncertain and erroneous examples iteratively, ensuring your models learn from the most challenging scenarios.
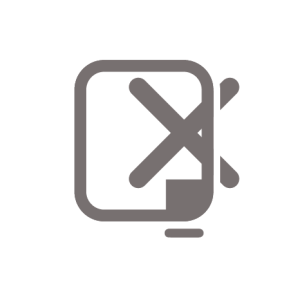
Swift Automation
GENIE performs automatic labeling and synthesis of examples, streamlining the process and boosting efficiency.
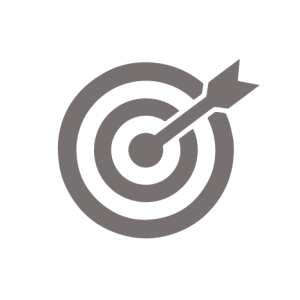
Precision Enhancement
By targeting rare and complex data slices and classes, GENIE elevates accuracy to new heights.
Enhancing Rare Class Detection for Autonomous Driving
- 45% improvement in rare-class detection
- Better performance in low-light and complex scenarios
- Faster AI retraining with minimal manual labeling
Improving Baggage Detection in Homeland Security
- 30% increase in detection accuracy for rare threats
- Lower false negatives, improving security screening
- Faster AI retraining with automated dataset curation
Detecting Debris on Runways for Airport Safety
- 60% reduction in false negatives for debris detection
- AI adapted to changing weather conditions
- Faster, automated AI retraining for improved safety